By
EJ Bowen
|
Date Published: December 10, 2023 - Last Updated December 12, 2023
|
Comments
Call centers are the core of the business, bridging the gap between brands and their audience. But as the digital curtain rises, customer expectations are no longer content with scripted dialogues. Enter generative AI, the behind-the-scenes wizardry rewriting the script of call center interactions. With an uncanny knack for predicting customer needs, generative AI isn't just reading lines – it's conducting an orchestra of personalized service that resonates with each individual's tune.
Imagine a call center that isn't merely reactive but intuitive. Generative AI paints this portrait, using a palette of data and empathy to craft conversations that feel as natural as a heart-to-heart chat. This shift isn't a magic trick but a response to a world where customers crave answers and an experience uniquely theirs. This article will discuss how call centers can adopt generative AI to predict customers' needs.
The Emergence of Generative AI in Call Centers
Significant shifts are happening in customer service, with call center interactions giving way to predictive and individualized customer engagement. This change is driven by the increasing influence of generative AI, combining natural language processing (NLP) and deep learning to decode, anticipate, and appropriately respond to customer queries.
Personalization Beyond Scripts
The versatility of generative AI surpasses the limitations of static applications. Traditional scripted conversations generate routine responses, which may fall short of the diverse spectrum of modern customer needs. Generative AI is an adaptable conversational partner that understands query context, sentiment, and specific preferences. This personalized approach surpasses prescribed norms to create genuine connections.
The Mechanics of Proactive Engagement
The capabilities of generative AI come from the combination of NLP and deep learning. Natural language processing imparts AI models with the ability to comprehend and interpret the complexities of human language, laying the groundwork for meaningful interactions. This is complemented by deep learning, which enables continuous learning from large datasets and refines responses over time.
Thus, generative AI enters the category of proactive engagement, in which it anticipates, comprehends, and responds to customer requirements before they emerge.
How JetBlue Is Using Generative AI
JetBlue, an airline based in the United States, is at the vanguard of using artificial intelligence (AI) and machine learning (ML) for both internal operations and revenue-generating products. JetBlue, in collaboration with technology provider ASAPP, has adopted a bundled generative AI solution to enhance the capabilities of its chat channel.
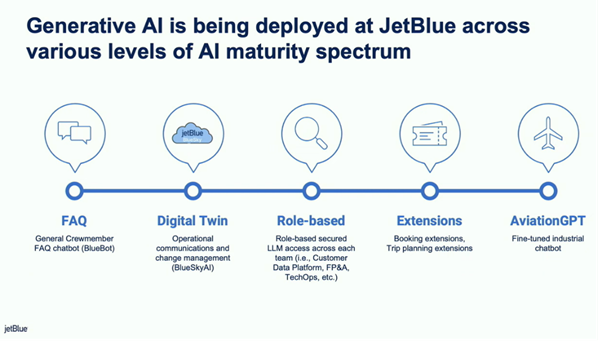
This implementation has resulted in significant efficiency gains in their contact center, reducing 280 seconds per conversation on average. This achievement represents an extraordinary savings of 73,000 hours of agent time for a single quarter. This enhanced efficacy enables JetBlue's agents to devote more time to resolving complex customer issues, thereby improving the quality of customer service offered.
Harnessing Data for Precise Predictions
According to Insight Enterprises, 81% of businesses have already established, implemented, or are in the process of establishing policies and strategies related to GenAI. Its potential lies in customer experience (CX). Approximately two-thirds of business executives anticipate the integration of GenAI to improve customer service within the next three years.
GenAI can rectify the joint misalignment between customer expectations and business priorities. Using sophisticated AI algorithms, digital avatars can swiftly respond to spoken or written communication, analyze real-time data, and identify customer needs.
GenAI is poised to improve every aspect of the consumer journey, including digital receptionists, sales personnel, and customer service agents.
John Lewis utilizes Salesforce's Einstein bot for answering basic questions, triages people, and uses large language models (LLMs) to improve website search and recommend more pertinent products to customers. They have yet to consider digital personas.
Ben Hull, service cloud manager at John Lewis, disclosed that adding Salesforce's chatbots to the retailer's CX arsenal has drastically reduced agent interactions but not customer satisfaction, as 98% of customers continue to give the retailer 5-star ratings.
Driving Operational Efficiency and Resource Optimization
In the complex choreography of call center operations, where customer questions are abundant and time is of the essence, generative AI acts as a conductor of efficiency and resource optimization. Its inherent ability to foresee and prioritize reduces wait times and queue lengths and orchestrates a symphony of AI-powered chatbots and virtual assistants.
Predictive analytics is one of the most notable features of AI. By analyzing historical call data, AI can predict patterns, such as peak call times or prevalent customer inquiries. This enables proactive changes, resulting in quicker response times and shorter wait times for callers. Customers are directed to the right agent for their chosen channel at any given time.
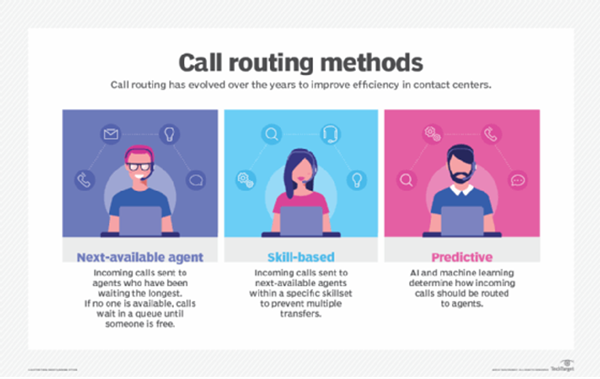
Generative AI enhances conventional call routing strategies by intelligently analyzing recipient data in real-time. It identifies the best available agent based on skills, performance metrics, and the caller's needs. Generative AI reduces call handling times and assures smoother call flows by eliminating the need for customers to repeat information.
Self-service options, such as automated responses to common queries, are also provided by Generative AI, enabling customers to receive quicker resolutions and reducing agent workload. The system's built-in analytics provide valuable insights into call center performance, enabling continuous improvement.
According to a McKinsey study, these tools can reduce call handling times by up to 50% and boost first-call resolution rates by up to 20%.
Empathy in the Digital Realm
Although much research has been devoted to training computer models to infer a person's emotional state based on their facial expression, this is not the most crucial aspect of human emotional intelligence. Significantly more essential is the ability to anticipate an individual's emotional reaction to events before they occur.
Researchers have created a computational model capable of predicting human emotions, incorporating social intelligence characteristics typically associated with the human "theory of mind." The model predicts emotions such as happiness, regret, and humiliation based on prisoner's dilemma game theory scenarios. The system predicts emotional responses according to a person's desires, expectations, and whether their actions are being observed. According to the researchers, the model outperformed its predecessors in predicting emotions, indicating significant advancements in emotional artificial intelligence.
Now, such models could be applied to the contact center environment. For example, NICE Enlighten is a collection of AI models embedded in the NICE CXone solution to give contact center administrators insights. NICE has also introduced Enlighten Actions, which employs generative AI to transform insight into action. Enlighten may detect, for instance, when consumer satisfaction is low in real-time. By notifying the supervisor, they can intervene and salvage the experience.
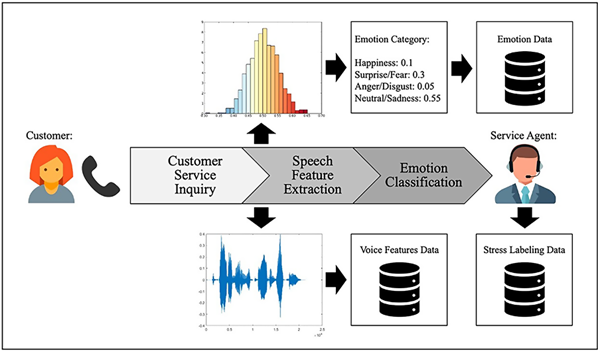
In addition, it may alert the administrator when an agent exhibits a specific behavior and report complaints as they occur. The latter use case can aid reporting efforts within a contact center.
Challenges and Future Directions
To navigate the landscape of predictive AI, it is essential to address potential pitfalls and ensure a balanced and impartial approach. Inadvertent bias may filter into generative AI's predictions, reflecting and reinforcing existing inequalities. For example, AI can racially profile people, leading to distress and incorrect decision-making.
Recognizing this, vigilant strategies are necessary for reducing biases. This process requires stringent data curation, diverse training datasets, and continuous monitoring. Transparency in AI decision-making and rigorous post-deployment audits provide a barrier against discrimination, fostering a technology that predicts and does so equitably and ethically.
* * * * *
The era of predicting customer needs with generative artificial intelligence has arrived, reshaping the call center landscape and paving the way for a new level of customer-centricity. As call centers transform into proactive problem-solvers with predictive capabilities, businesses have a unique opportunity to exceed consumer expectations.
The combination of technology and empathy propels the call center experience into uncharted territory, where each interaction is an opportunity to anticipate, meet, and exceed expectations.